As artificial intelligence (AI) and machine learning (ML) continue to evolve, the demand for specialized hardware to handle complex computations grows exponentially. Enter the NVIDIA H200 Tensor Core GPU, a groundbreaking graphics processing unit designed to accelerate the most demanding AI workloads. With unprecedented computational power, energy efficiency, and scalability, the H200 Tensor Core GPU is set to play a pivotal role in the future of AI research, deep learning, and high-performance computing (HPC).
In this article, we’ll explore the features, benefits, and potential applications of the NVIDIA H200 Tensor Core GPU, while also comparing it to other GPUs in NVIDIA’s lineup, such as the A100 and H100.
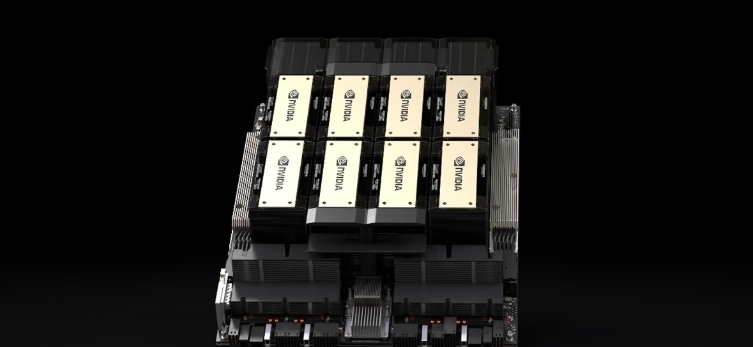
What is the NVIDIA H200 Tensor Core GPU?
The NVIDIA H200 Tensor Core GPU is part of NVIDIA’s latest generation of AI-focused hardware, designed to optimize the performance of machine learning and deep learning tasks. It is engineered to address the increasing complexity of AI models and the vast datasets they require. As part of NVIDIA’s H100 series, the H200 offers enhanced performance and efficiency, making it ideal for both training large neural networks and performing real-time inference in AI-powered applications.
One of the standout features of the H200 is its Tensor Core technology, which is purpose-built to accelerate matrix multiplication, a key operation in machine learning algorithms. Tensor Cores enable the GPU to perform calculations with higher throughput and lower latency compared to traditional GPU cores, making them a critical component for AI workloads.
Key Features of the NVIDIA H200 Tensor Core GPU
- Advanced Tensor Core Architecture The Tensor Cores in the H200 are optimized for mixed-precision AI workloads, meaning they can handle both low-precision calculations (such as FP16 or BFLOAT16) and higher-precision calculations (such as FP32 or TF32). This capability allows the H200 to deliver faster training and inference speeds while maintaining high accuracy, essential for deep learning tasks.
- Massive Memory Bandwidth The H200 features a high-bandwidth memory system, ensuring that large datasets can be processed quickly and efficiently. With 48GB of high-bandwidth memory (HBM2), the H200 delivers the bandwidth needed to accelerate demanding AI and ML models, particularly those with high data throughput requirements.
- Multi-Instance GPU (MIG) Technology MIG technology allows the H200 to partition the GPU into multiple smaller, independent instances. This enables the simultaneous training of multiple AI models on a single H200 GPU, improving resource utilization and reducing infrastructure costs. MIG is particularly beneficial in cloud-based AI services where multiple users or organizations need access to GPU resources.
- Enhanced AI Optimizations The H200 is designed for a wide range of AI tasks, including deep learning, neural network training, and model inference. Its architecture is fine-tuned for key AI frameworks like TensorFlow, PyTorch, and MXNet, ensuring seamless integration and performance optimization.
- Scalability and Efficiency The H200 offers scalability to meet the needs of both small-scale and enterprise-level AI deployments. Its architecture is designed to work seamlessly in multi-GPU setups, allowing data centers and supercomputing environments to scale up AI workloads across hundreds or thousands of GPUs. Additionally, the H200 is highly energy-efficient, delivering superior performance per watt, which is critical for reducing operating costs and environmental impact in large AI infrastructure setups.
Performance Benchmarks: NVIDIA H200 vs. A100 and H100 GPUs
When comparing the H200 to NVIDIA’s previous-generation A100 and H100 GPUs, the H200 outperforms both in several key areas, including raw computational power and energy efficiency.
- NVIDIA A100 GPU: The A100 was a breakthrough in AI performance, leveraging Ampere architecture for high throughput in both training and inference tasks. However, the H200 offers improved tensor core performance, higher memory bandwidth, and better scalability, making it more suitable for training next-generation AI models.
- NVIDIA H100 GPU: The H100 Tensor Core GPU, based on the Hopper architecture, was designed for cutting-edge AI workloads, particularly large-scale deep learning and AI training. The H200 takes this a step further by improving multi-GPU scalability, memory capacity, and real-time inference, making it a more versatile solution for diverse AI applications.
In terms of performance metrics, the H200 Tensor Core GPU offers significant improvements over both the A100 and H100, particularly in tasks requiring high-precision matrix multiplication and large-scale data processing.
Use Cases and Applications of the NVIDIA H200 Tensor Core GPU
The NVIDIA H200 Tensor Core GPU is poised to accelerate a wide range of applications in AI, machine learning, and high-performance computing. Some key use cases include:
- Deep Learning and Neural Network Training With its superior processing power and Tensor Core optimizations, the H200 is ideal for training deep learning models, especially those involving large datasets and complex architectures like transformers or GANs (Generative Adversarial Networks). It can significantly reduce training times for state-of-the-art AI models.
- AI Inference at Scale The H200 is optimized for running AI inference in real-time, making it well-suited for applications like autonomous vehicles, robotics, and natural language processing. Its high bandwidth and low latency ensure fast and accurate results, even in mission-critical systems that require real-time decision-making.
- Medical AI and Genomics In fields like healthcare, where large datasets (such as medical imaging and genomic data) are common, the H200 provides the computational power needed to accelerate the analysis and interpretation of this data. AI models in medical imaging (e.g., MRI scans or X-rays) can be trained faster, leading to quicker diagnoses and better patient outcomes.
- Autonomous Systems The H200’s ability to handle complex AI algorithms in real-time makes it ideal for autonomous systems, such as self-driving cars, drones, and robotics. These applications require high-throughput processing and low-latency responses, both of which the H200 excels at providing.
- AI-Driven Natural Language Processing (NLP) Training large NLP models, like GPT-3 or BERT, requires immense computational power. The H200’s Tensor Core architecture makes it particularly well-suited for training language models at scale, enabling faster development and deployment of advanced NLP systems.
Conclusion: The Future of AI with the NVIDIA H200 Tensor Core GPU
The NVIDIA H200 Tensor Core GPU represents the next evolution in AI hardware, offering exceptional performance for machine learning and deep learning applications. With its advanced Tensor Core architecture, massive memory bandwidth, and scalability, the H200 is poised to become a key component in powering the future of AI.
Whether you’re training cutting-edge AI models, performing real-time inference, or deploying AI-driven solutions in industries like healthcare, automotive, or finance, the H200 delivers the speed, efficiency, and scalability needed to drive innovation.
As the demand for AI capabilities continues to grow, the NVIDIA H200 will play a central role in enabling the next wave of AI advancements, pushing the boundaries of what artificial intelligence can achieve across a variety of fields.